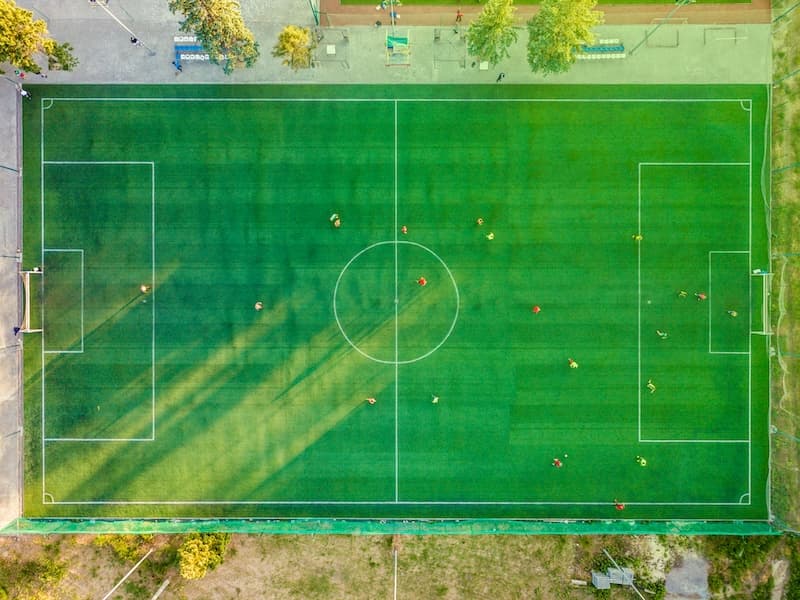
What is a Soccer Data Analyst? A Complete Guide
Discover the role of a soccer data analyst, their responsibilities, tools, and impact on modern soccer. Learn how to become one and the challenges they face.
If you've ever wondered how soccer clubs make better decisions on the pitch, you're probably familiar with the work of a soccer data analyst.
In recent years, data analysis has transformed the game of soccer, giving teams an edge by uncovering insights that go beyond what meets the eye. Whether you're aspiring to enter this growing field or just curious about the role, this blog post will explain what a soccer data analyst does, why they are important, and how you can become one.
Understanding the Role of a Soccer Data Analyst
A soccer data analyst is a professional who collects, processes, and interprets data related to the game. They play a key role in helping teams make data-driven decisions, from tactics and player recruitment to injury prevention and scouting. Their job is to transform the vast amount of data generated during training sessions, games, and scouting activities into meaningful insights that coaches, managers, and decision-makers can use to improve team performance.
Soccer data analysts are often involved in all aspects of a team's performance. They work closely with coaches to develop game strategies based on statistical insights, assist scouts in identifying talent that fits the team's needs, and even collaborate with medical staff to track player health and prevent injuries. This multifaceted role makes them an integral part of any modern soccer club's success.
Key Responsibilities of a Soccer Data Analyst
The responsibilities of a soccer data analyst typically include:
Data Collection: Gathering data from different sources, such as match events, player statistics, and GPS tracking systems. This includes both publicly available data and proprietary data collected through sensors and video analysis. Data collection is the foundation of any analysis, and a good soccer data analyst knows how to source reliable and comprehensive data.
Data Cleaning and Processing: Ensuring data quality by cleaning raw data, correcting errors, and organizing it in a format suitable for analysis. Raw data is often messy and inconsistent, and data analysts must standardize it to make it usable. This step is crucial, as even the most sophisticated analysis can be rendered meaningless by poor data quality.
Data Analysis: Using statistical models, coding, and machine learning tools to analyze player performance, team tactics, and more. This includes expected goals (xG), possession metrics, and defensive performance indicators. Analysts may also use advanced machine learning techniques to create predictive models that forecast match outcomes or player performance.
Reporting and Visualization: Communicating key insights through dashboards, reports, and visualizations to stakeholders like coaches and analysts. Tools like Python, R, and Power BI are commonly used for this purpose. Effective communication is key, as the insights generated must be easily understood by coaches and staff who may not have a background in data science.
Scouting and Recruitment: Assisting the scouting team by using data to identify new talent that matches the team's tactical needs. This involves analyzing player performance data from other leagues to find undervalued players who have the potential to succeed in the team's system. Data analysts often use metrics like expected assists, progressive passes, and defensive actions to evaluate potential recruits.
In-Game Analysis: Providing live data analysis during matches. Many soccer data analysts are part of the match-day staff, providing real-time insights that help coaches make tactical adjustments on the fly. This could involve identifying weaknesses in the opposing team's formation or highlighting areas where the team can exploit space.
Tools and Techniques in Soccer Data Analysis
Soccer data analysts rely on various tools to perform their job effectively:
Data Analysis Tools: Python and R are popular for statistical analysis, while SQL is often used for managing large data sets. Python, in particular, is highly versatile, allowing analysts to build custom models, automate data processing, and create visualizations. R is known for its strong statistical capabilities, making it a favorite for complex statistical analysis.
Tracking and Event Data: Analysts use tracking data to measure player movement and event data to understand what happens during matches (e.g., passes, tackles, shots). Tracking data is collected using cameras or GPS systems, providing detailed information about player positioning, speed, and acceleration. Event data, on the other hand, records every action that takes place during a game, such as passes, shots, and fouls.
Machine Learning: Machine learning techniques are increasingly used to gain deeper insights. For example, clustering algorithms can group players with similar styles, while regression models can predict player performance or injury risk. Analysts use machine learning to create predictive models that can forecast match results, player fatigue levels, and even transfer market values.
Visualization Software: Software like Tableau or Power BI is used to create visual reports that are easy for coaches and staff to understand. Visualization is an essential skill, as it helps translate complex data into actionable insights. Analysts often create heatmaps, passing networks, and player dashboards that simplify data interpretation for non-technical stakeholders.
Why Are Soccer Data Analysts So Important?
The impact of a soccer data analyst can be seen in how modern soccer has evolved. Teams like Liverpool FC and Manchester City have famously leveraged data analysis to stay ahead of the competition. Here are some reasons why soccer data analysts are vital:
Optimizing Performance: Analysts can identify patterns that reveal a team's strengths and weaknesses, enabling more strategic game plans. For example, analysts might discover that a team is particularly vulnerable to counter-attacks, prompting the coaching staff to adjust their defensive shape.
Preventing Injuries: Data from training sessions can be analyzed to monitor player workloads, reducing the likelihood of injuries. Analysts work closely with sports scientists and medical staff to ensure players are not overworked and to predict injury risk based on workload metrics. This helps maintain player fitness and availability throughout the season.
Informed Recruitment: By identifying undervalued talent, data analysts can help clubs maximize their budget and bring in players who fit their playing style. Moneyball-style recruitment, which focuses on finding players with specific metrics at a lower cost, has been popularized by several clubs. Analysts can identify players whose statistical profiles match the team's needs, even if they are not well-known.
Tactical Insights: Data analysis provides insights into opposing teams, helping coaches develop tactical plans. By studying data on opponents, analysts can identify tendencies, such as how a team defends set-pieces or which players are most prone to losing possession. This information can be used to exploit weaknesses and enhance game strategies.
Real-Life Examples of Soccer Data Analysis
To illustrate the importance of soccer data analysis, let's look at some real-life examples:
Liverpool FC and Expected Goals (xG): Liverpool FC has been a pioneer in using data analytics, particularly in understanding expected goals (xG). By focusing on xG, Liverpool has been able to better evaluate the quality of their chances and improve their attacking efficiency. This has contributed to their success in the Premier League and Champions League.
Brentford FC and Data-Driven Recruitment: Brentford FC is well-known for its data-driven recruitment strategy. The club uses statistical models to identify undervalued players in lower leagues, helping them compete with bigger teams despite having a smaller budget. Their approach has been instrumental in their promotion to the Premier League.
Manchester City's Use of Tracking Data: Manchester City uses tracking data to analyze player positioning and movement. This allows them to optimize their playing style, ensuring that players are in the best possible positions to retain possession and create scoring opportunities. Tracking data has been key in helping City maintain their high-possession, attacking style of play.
How to Become a Soccer Data Analyst
If you're interested in becoming a soccer data analyst, here are some steps to follow:
Learn the Fundamentals of Soccer: Understanding the game inside and out is crucial. Learn about tactics, formations, and the roles of different positions. This knowledge will help you make sense of the data you work with and communicate effectively with coaches and staff.
Acquire Technical Skills: Knowledge of statistics, coding languages like Python, SQL, and data visualization tools are essential. You should also understand soccer-specific metrics, such as xG and passing networks. Courses in data science, sports analytics, and statistics can provide you with the necessary foundation.
Build a Portfolio: Practice analyzing publicly available soccer data and create visualizations or reports that demonstrate your skills. Your portfolio should showcase your ability to analyze data, generate insights, and communicate findings. You can use data from sources like StatsBomb or Opta, or even work with data from amateur leagues.
Network and Stay Informed: Connect with others in the industry, attend conferences, and stay up-to-date with the latest trends in soccer analytics. Twitter, LinkedIn, and online forums are great places to connect with professionals in the field. Engaging in the soccer analytics community can open up opportunities and help you learn from others.
Gain Practical Experience: Look for internships or volunteer opportunities with soccer clubs, especially at lower levels. Many clubs are beginning to see the value of data analysis, and smaller clubs may be willing to give you hands-on experience. This practical exposure is invaluable and can help you land a full-time role in the future.
Challenges Faced by Soccer Data Analysts
While being a soccer data analyst is rewarding, it also comes with its challenges:
Data Quality: The quality of data can vary, especially at lower league levels. Missing or inaccurate data can make it difficult to draw reliable conclusions. Analysts need to be skilled in handling imperfect data and making informed judgments.
Integration with Coaching Staff: Translating complex data into actionable insights for coaches can be challenging. Not all coaches are comfortable with data, and analysts must find ways to present information in a way that is accessible and useful. Building trust with coaching staff is essential to ensure that data-driven recommendations are implemented.
Time Constraints: During the season, analysts often have limited time to process and analyze data before the next match. The fast-paced nature of soccer means that insights need to be generated quickly, often under pressure. Analysts must be efficient and prioritize the most important information.
Balancing Qualitative and Quantitative Analysis: Soccer is a complex sport that involves both quantifiable metrics and qualitative elements, such as player mentality and teamwork. A good soccer data analyst must balance data-driven insights with an understanding of the qualitative aspects of the game.
Conclusion
Soccer data analysts are revolutionizing the way the game is played, providing insights that improve team strategies, player performance, and decision-making. With a mix of technical skills, love for the game, and a desire to draw insights from data, a career in soccer data analytics can be both rewarding and impactful.
Whether you're a die-hard soccer fan or someone interested in data science, becoming a soccer data analyst could be your opportunity to be part of the beautiful game in a unique and exciting way. The field of soccer analytics is growing rapidly, and there has never been a better time to get involved.
Interested in learning more about soccer analytics? Subscribe to our newsletter or check out our in-depth courses on how to analyze soccer data and kick-start your journey into sports analytics. Learn how to work with real-world soccer data, build predictive models, and visualize insights that can change the game.